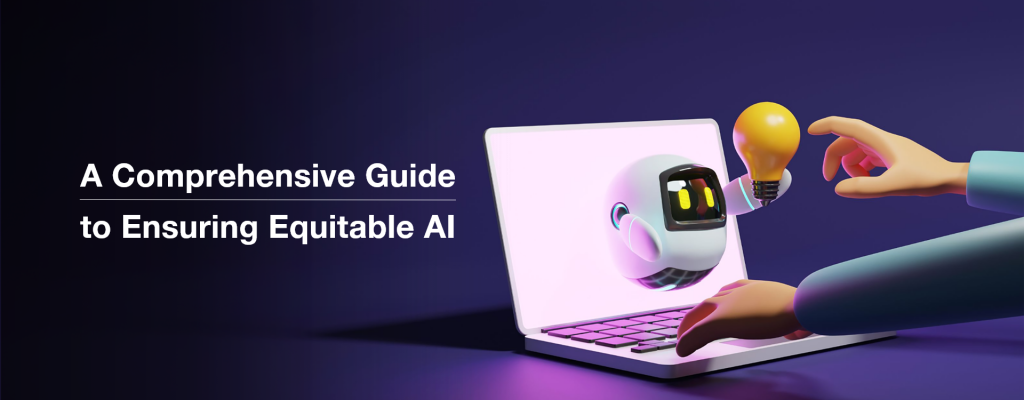
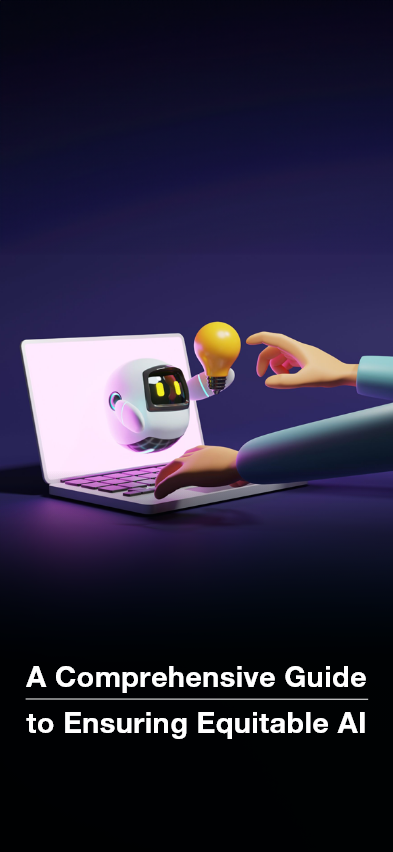
Fairness is a fundamental aspect of any machine learning system. When developing AI models, it is crucial to consider the potential biases that can arise from the data used to train these models. Biases can emerge from historical patterns of discrimination, underrepresentation, or unequal treatment in the data. If these biases are not addressed, they can lead to unfair outcomes for certain individuals or groups.
Ensuring fairness in machine learning is not only an ethical imperative but also a legal requirement in many jurisdictions. Discriminatory AI systems can lead to harmful consequences, perpetuating existing inequalities and excluding marginalized communities. Fairness is essential to build trust in AI systems and to ensure that they benefit all individuals and groups equally.
Machine learning fairness frameworks provide a systematic approach to identify, measure, and mitigate biases in AI systems. By using these frameworks, developers can assess the fairness of their models and take appropriate steps to address any potential biases.
There are several machine learning fairness frameworks available that we have discussed in our previous blog that can help developers promote fairness in their AI systems. Let’s explore some of the most widely used ones:
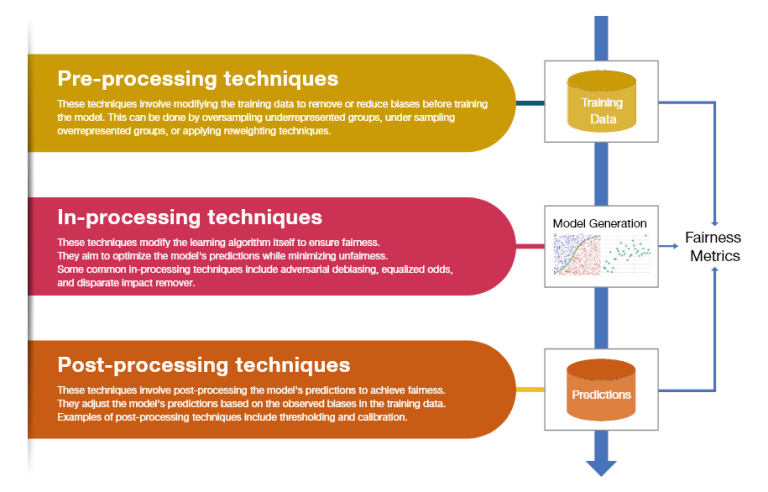
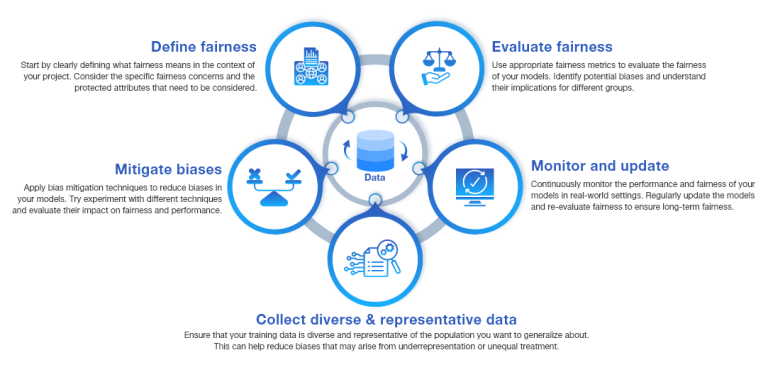
- Lack of consensus on fairness definitions: Fairness is a complex and multifaceted concept, and there is no universally agreed-upon definition of fairness. Different stakeholders may have different perspectives on what constitutes fairness, which can make it challenging to design and implement fair AI systems.
- The trade-offs between fairness and performance: Achieving fairness in machine learning models often involves trade-offs with other desirable properties, such as accuracy or efficiency. Striking the right balance between fairness and performance can be challenging, and developers need to carefully consider the implications of their choices.
- Lack of transparency and interpretability:Some fairness techniques, especially those involving complex algorithms or post-processing steps, can make models less interpretable. Lack of transparency can raise concerns about accountability and trust in AI systems.
- Ongoing biases in data collection: Bias in machine learning models can often be traced back to biases in the training data. Addressing these biases requires addressing the underlying societal biases that are reflected in the data collection process.
Ensuring fairness in machine learning is crucial to building a future of equitable AI. As AI becomes more pervasive in various industries and applications, it is essential to address biases and promote fairness in AI systems. Machine learning fairness frameworks provide valuable tools and methodologies to achieve this goal.
By evaluating fairness, mitigating biases, and implementing fairness in machine learning projects, developers can build AI systems that are more inclusive, transparent, and accountable. Fairness frameworks empower developers to make informed decisions about the fairness of their models and take appropriate actions to promote equity. As we continue to advance in the field of AI, it is important to keep fairness at the forefront of our discussions and actions. By working together to address biases and promote fairness, we can shape a future where AI benefits all individuals and groups equally. Let’s embrace the power of machine learning fairness frameworks and build a future of equitable AI.