Build a Strategy for Enterprise-Ready Generative AI
The time has come to get started
Generative AI, especially large language models (LLMs) like ChatGPT, Bard,
and Bin, will fundamentally change the way we do knowledge work. These
technologies can automate many of the tasks that are currently done by
humans, which will lead to significant cost savings.
Companies that are able to adopt generative AI early will gain a competitive
advantage. Those that wait will be left behind.
Now is the time to start experimenting with generative AI use cases. It is also important
to start developing a generative AI strategy. This strategy should help you to:
- Turn generative AI use cases into reality quickl
- Safeguard against risk
- Be future proof for further AI developments
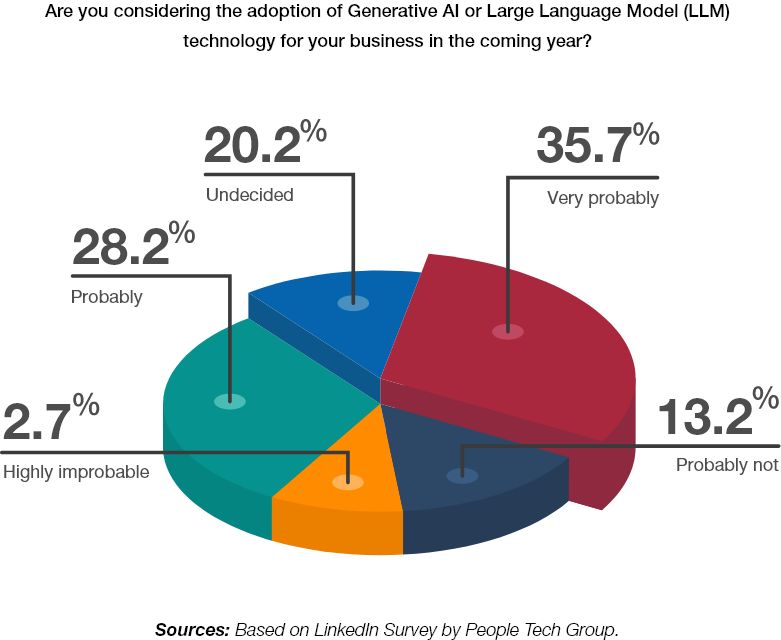
The Potential of
Generative AI is truly Tangible
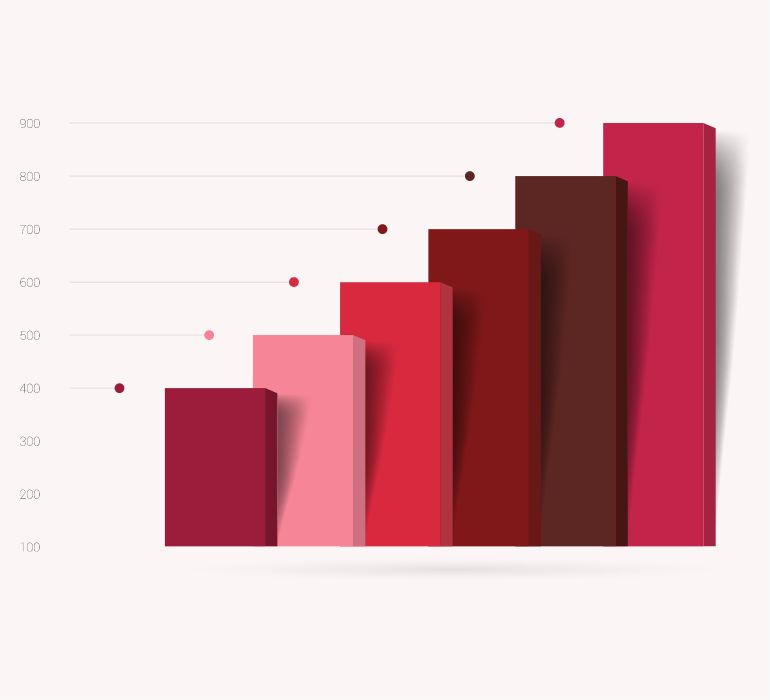
In David Graeber’s book “Bullshit Jobs: A Theory, “he highlights a pervasive issue in modern organizations: the mundane and uninspiring nature of work. Graeber coins the term “bullshit jobs” to describe these roles that, while deemed essential, often fail to
tap into the unique human abilities of creativity, intelligence, and judgment.
One potential solution to this problem is the use of Generative AI. By automating these monotonous tasks and applying similar AI-driven methods to various other value-enhancing activities, both employees and organizations can benefit. It’s essential for you, your colleagues, groups, and executives to understand that a Generative AI strategy goes far beyond just implementing chatbots. The ultimate objective is to imbue a wide range of business processes across your entire organization with human-like cognitive abilities. This approach can revolutionize the way work is done, leading to greater efficiency, innovation, and job satisfaction Now is the time to start experimenting with generative AI use cases. It is also important to start developing a generative AI strategy. This strategy should help you to:
The main idea is to make processes in your business smarter,
like how humans think.
This change is not just about saving money, but also about finding new ways to make money and giving customers better experiences. For a long time, AI developers wanted this, and now, with the latest AI technology, it’s becoming possible.
The hype around
Generative AI is justified
Generative AI models’ capabilities have surpassed even their developer’s expectations. This technology is leading the way us into a new era.
We are still in the early stages of adopting Generative AI in the enterprise. Businesses are rightly risk-averse when it comes to adopting new technologies. They need to fully
understand the performance and capabilities of Generative AI before they can adopt it widely.
That said, the transformation of businesses by Generative AI is inevitable. The technology is too powerful and too versatile to be ignored. In the coming years, we will see Generative AI being used in a wide variety of applications, from product design to customer service to marketing.
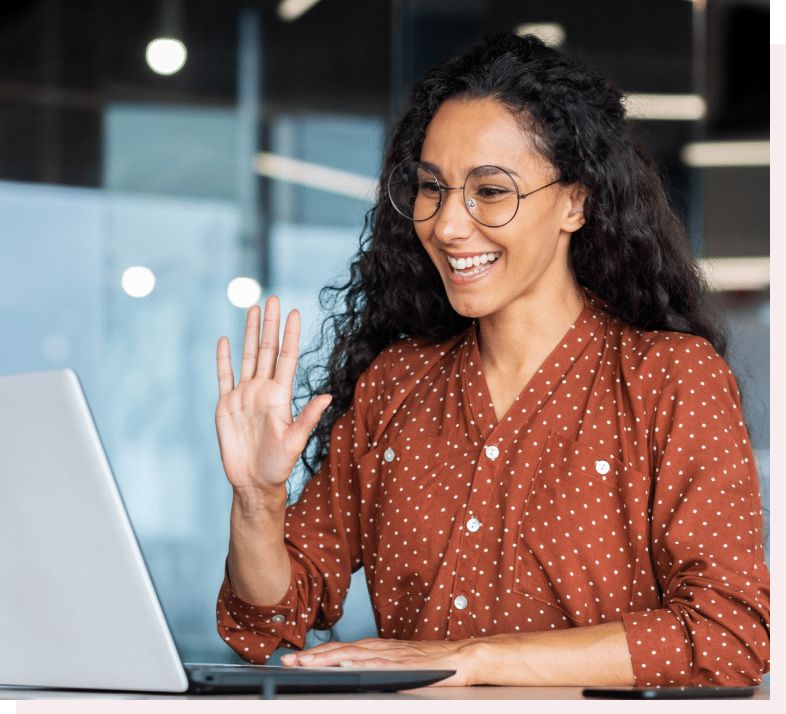
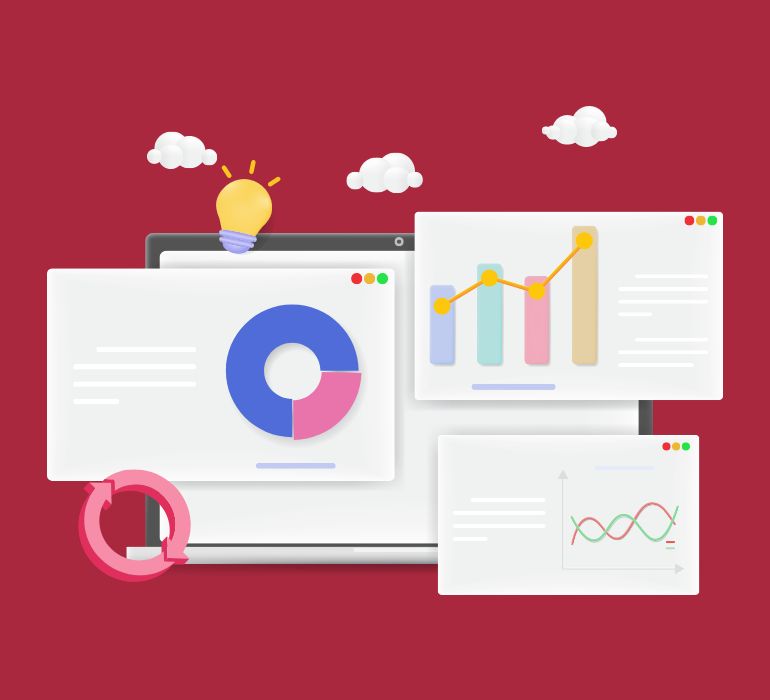
What Sets Generative AI Apart?
For many years, people have been working on and using technologies like machine learning and artificial intelligence. It’s interesting to note that a crucial technology called Generative AI, which relies on something called “transformer models, ” has been around since 2017.
The big AI models we see today, often called “foundation models,” are important because they serve as the building blocks for other AI systems. They’re quite different from what we had before. These models are huge and use a new kind of architecture. This is possible because of all the research on neural networks and the increased availability of powerful cloud computing.
One of the important things about these foundation models is that they come pretrained. What’s groundbreaking here is that you can achieve really impressive results even with smaller sets of data when you use these models. This has the potential to make AI more accessible to a wider range of people.
- Generate human-quality text in a variety of styles and languages
- Extract information and summarize complex text in a way that is easy to understand.
- Outperform traditional NLP models in tasks such as sentiment analysis and entity extraction.
These capabilities are made possible by the fact that foundation models are trained on massive datasets of text and code. This allows them to learn the statistical relationships between words and phrases, which gives them a deep understanding of language.
Foundation models are still under development, but they have the potential to revolutionize the way we interact with computers. They could be used to create more
natural and engaging chatbots, to improve the accuracy of machine translation, and to
develop new ways of generating creative content.
Today’s foundation models are more powerful and versatile than ever before. They can:
Here are some specific examples of how foundation models are being used today:
- ChatGPT is a chatbot powered by a foundation model that can hold conversations that are indistinguishable from those with a human.
- Bard is a large language model that can generate text, translate languages, write different kinds of creative content, and answer your questions in an informative way
- Bing uses a foundation model to improve the accuracy of its search results and to personalize its recommendations.
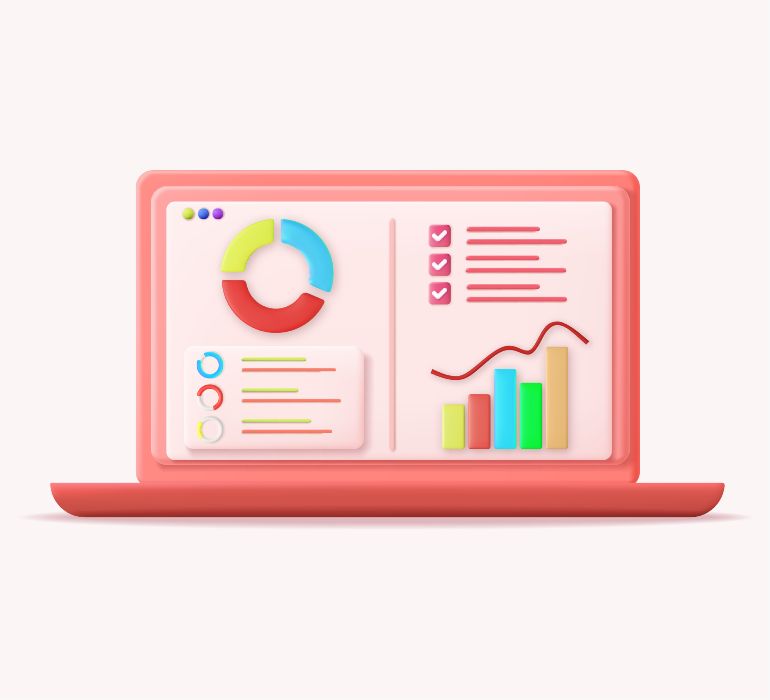
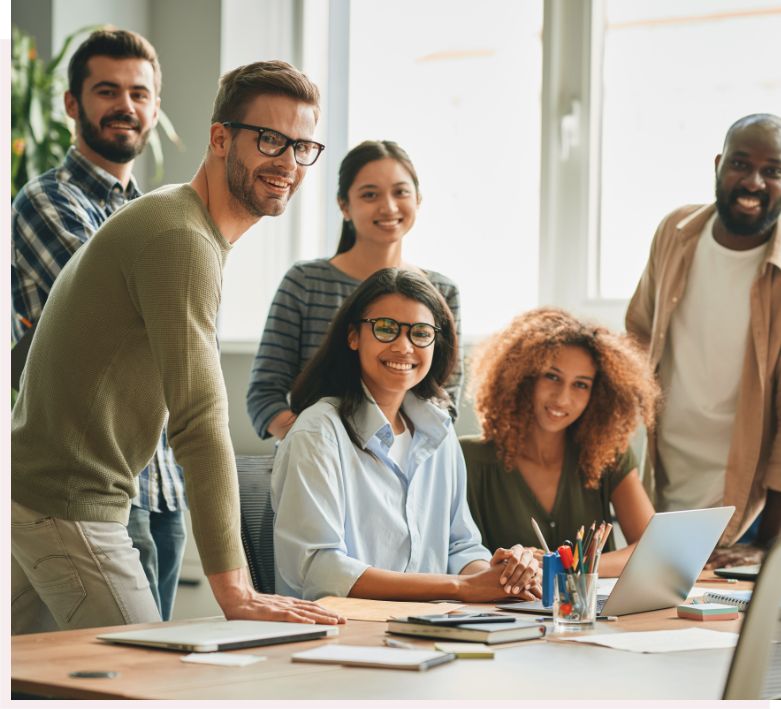
These are just a few examples of the many ways that foundation models are being used today. As these models continue to develop, we can expect to see even more innovative and exciting applications in the years to come.
The above examples in the original text all involve the generation of text, such as product descriptions, blog posts, and social media ads. Automated generation is not limited to text. It is also being used to generate images and videos, and the technology for doing this is rapidly improving. Businesses are starting to use automated generation to create marketing materials that are tailored to their target audience.
These models do not always produce the same output when given the same input. This is because they are trained on large amounts of data, and the data is not always perfectly consistent. These models make use of randomness in their training process. This randomness can introduce variability into the output of the models, which is why they do not always produce the same output when given the same input.
The stochastic nature of these models can make it difficult to use them in certain situations. For example, if a business needs to generate many marketing materials that are
all the same, then it may not be possible to use a stochastic model.
Understanding the Dual Tiers of Generative AI
Early AI was like a horse-drawn carriage, but generative AI models are like automobiles. They have the potential to transform businesses in ways that we can’t
even imagine.
There are two main ways that generative AI can be used:
- Moonshots: Generative AI can be used to create radically new business capabilities that have the potential to revolutionize the industry. For example, generative AI could be used to create new drugs, design new products, or develop new marketing campaigns.
- Mundane: Generative AI can also be used to improve existing business processes and decisions. For example, generative AI could be used to improve customer service, optimize supply chains, or automate financial reporting
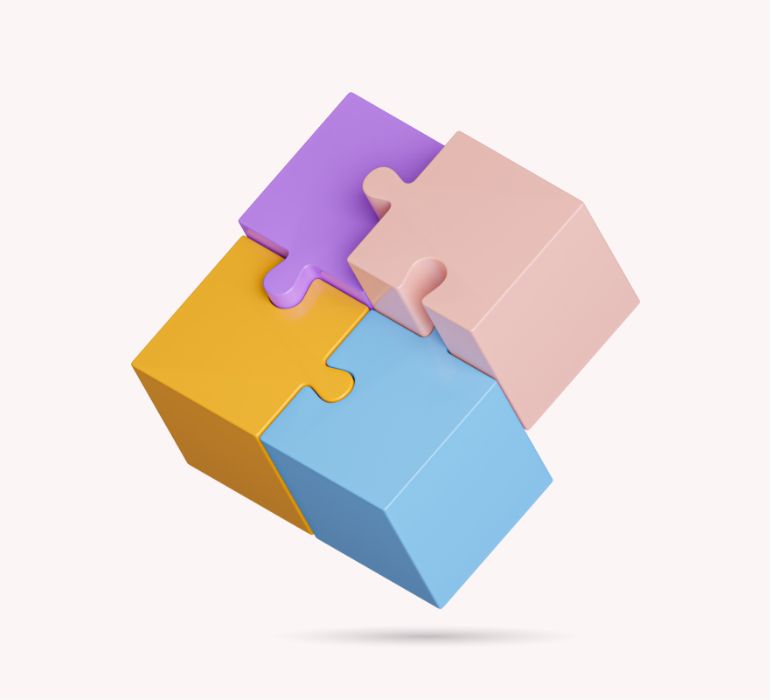
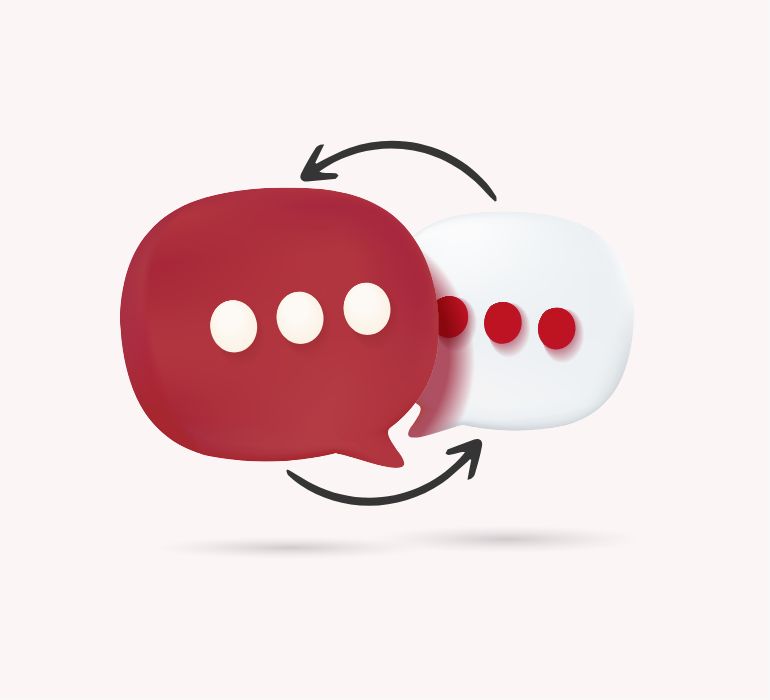
Finding the Right Balance Between Moonshots and Mundane in AI
When considering the application of advanced AI in companies, one prominent concept is the creation of a highly intelligent chatbot. Imagine a chatbot that’s always ready to provide information about the company’s current happenings and even predict future developments. Can such a chatbot be successful? Well, it’s a possibility, but there are several factors to ponder, and doubts linger about whether people would fully trust such a system.
Another aspect of AI’s potential impact on businesses often goes overlooked. It involves simplifying everyday tasks through AI. Under this approach, companies would select different AI tools for various tasks, taking into account factors like their effectiveness, cost, and privacy. Teams would then utilize these tools to address their specific challenges. This democratizes technology, empowers individuals, and has the potential to significantly enhance productivity as teams devise their solutions.
Therefore, it’s essential to consider both avenues.
While pursuing ambitious AI projects is valuable, what’s even more critical is for companies to leverage AI to enhance routine tasks throughout the entire organization. Striking the right balance between allowing teams autonomy and maintaining some oversight is imperative.
Making Generative AI Work for Your Business
If you want to tap into the benefits of generative AI for your business, it’s vital to grasp its proper usage.
Take a look at these pointers to effectively apply generative AI to your business:
ENTERPRISE DATA
When we "fine-tune" an LLM or another Generative AI model using our own data, it allows the model to give very specific and accurate answers that are exactly what our business needs. This can be used for many things, like making it easier to review documents or creating smart systems to manage our knowledge.IMPROVING PROMPTS
We're finding out how these models react to different prompts. We want to create tools that help people send better prompts to make the models more useful. Once we figure out what works best, we'll make it standard practice within the enterprise.ENHANCING PREDICTION
LLMs struggle with the math and statistics needed for predictive models. When you combine an LLM's language skills with the strong math of a predictive model, you can create tailored content that influences actions effectivelyHUMAN REVIEW & INPUT
The models won't always get it right, and not all their responses will be suitable. Offering feedback in a controlled manner will enhance the model and establish a scalable method for preserving institutional knowledge.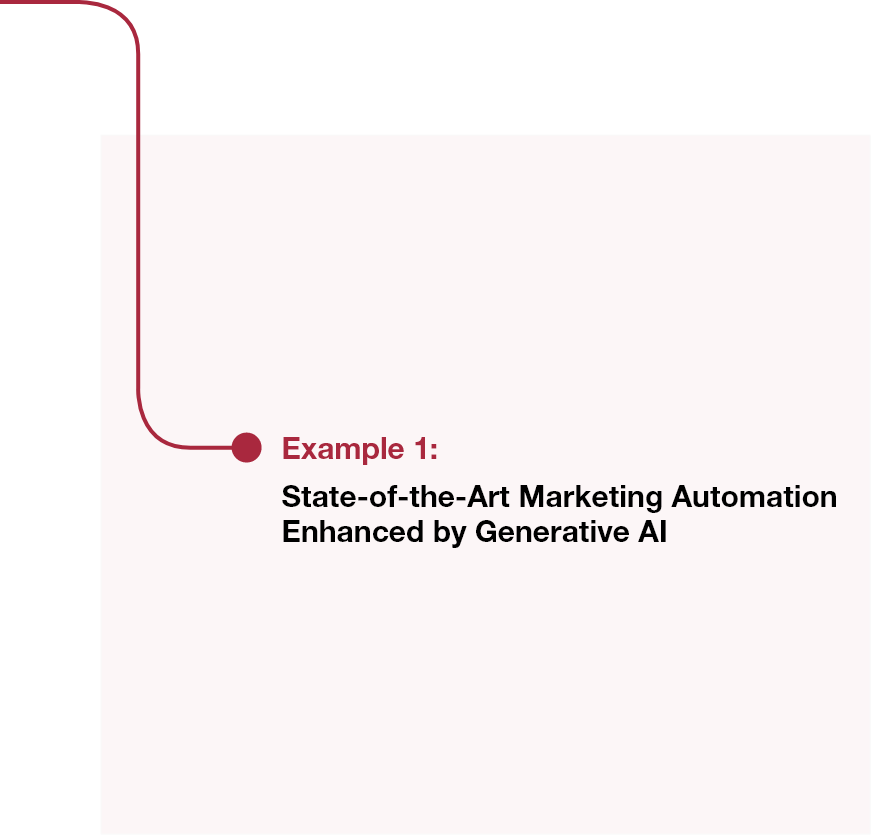
- Pre-Generative AI Era: In the era prior to Generative AI, a predictive model would create product recommendations based on a customer's known demographic information and purchase history. These recommendations were delivered through pre-made email templates, but frequently encountered issues such as getting caught in spam filters or being disregarded by consumers due to their formulaic nature
- With Generative AI Advancements: In the modern age of Generative AI, the same product recommendations are seamlessly integrated into highly personalized, multilingual, and consistently unique conversations with individual customers. As an illustration, a fashion retailer could craft a virtual personal stylist tailored to each customer, complete with an appropriate tone and style. This approach allows customers to engage with the virtual stylist, fostering a connection and ultimately boosting sales.
- Key Consideration: It's essential to note that hyper-personalized content can backfire if it reaches the wrong audience. Therefore, it's imperative to ensure that your segment building involves the right stakeholders. Start by conducting live tests of this solution with your employees and subsequently with trusted customers to ensure it generates the desired impact. This strategic approach will help you make headlines for all the right reasons.
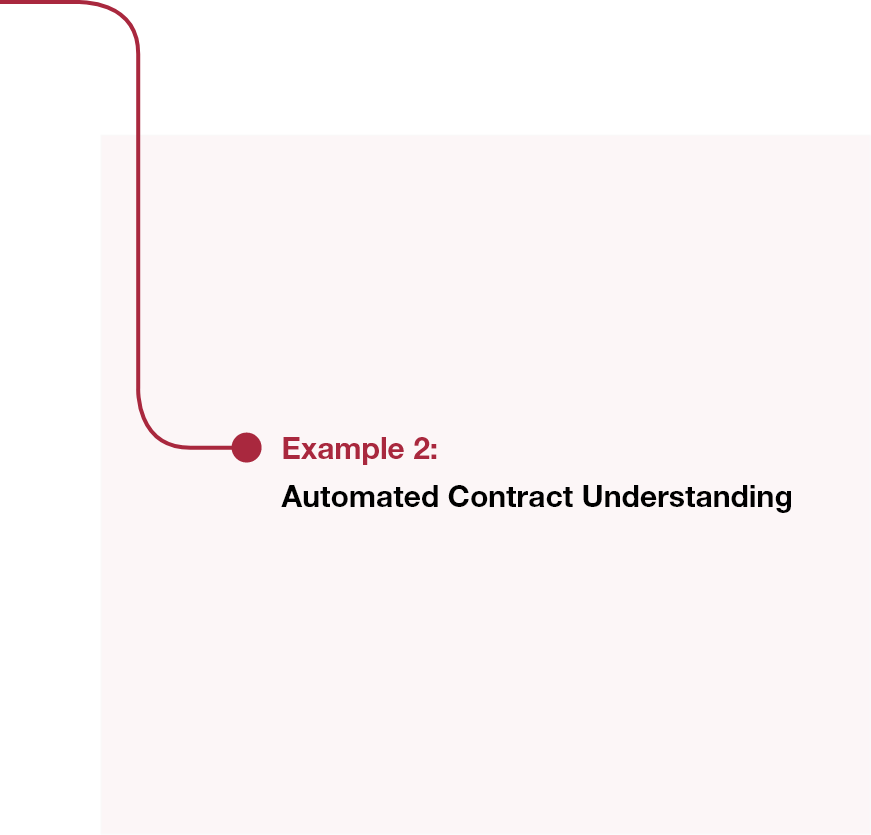
- Before Generative AI: When companies wanted to make their sales and purchasing processes more efficient, they used rule-based methods that combined different language analysis techniques to sort and understand contracts. Contracts come in all sorts of languages and formats, making this process difficult. It led to the creation of complex, customized rules that were delicate and needed constant attention from experts.
- With Generative AI: Now, organizations can employ a Language Model (LLM) to automatically extract important parts of contracts, turning messy documents into structured, organized data. With this data, they can also automate tasks like calculating complex financial risks. In the past, finding and extracting this data would require an expensive lawyer with specialized skills. LLMs open the door to scaling up this specialized knowledge.
- Key consideration: Even though AI is powerful, it can still make mistakes. Make sure a human is in charge and checks the AI's work thoroughly. This not only helps improve the system but also reduces the chances of errors and risks.
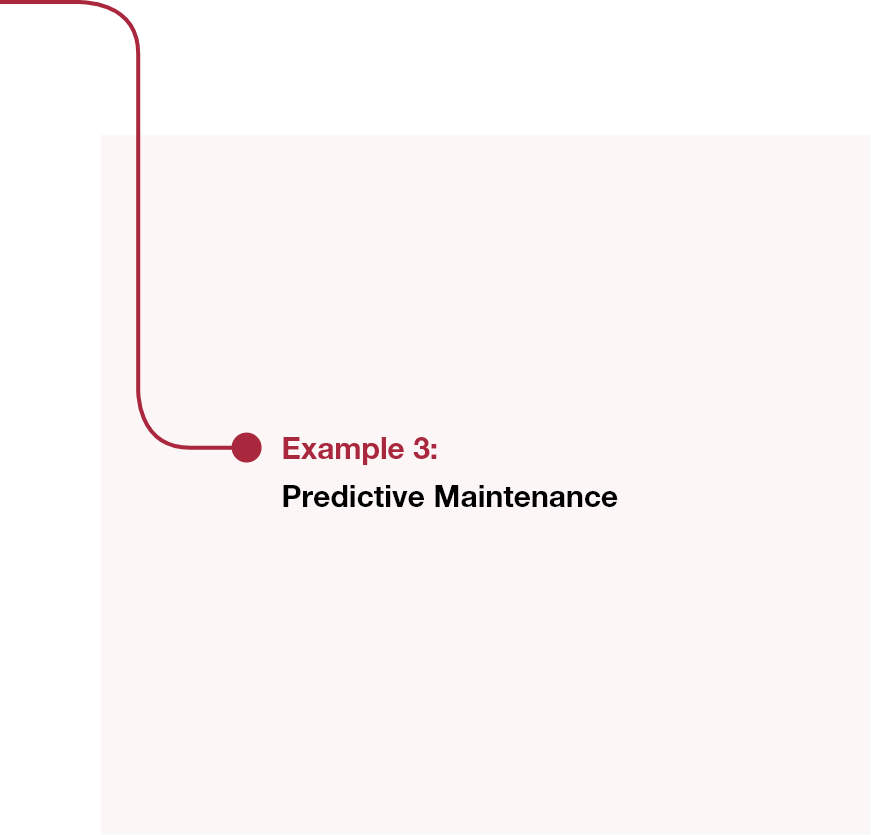
- Before Generative AI: Previously, a predictive maintenance model would create a static list of machines for daily inspection in a factory. This list was given to a technician as a fixed report on a clipboard or digital device. The technician would then inspect the machines but lacked context and instant feedback.
- With Generative AI: Today, thanks to Generative AI, the process has improved. Instead of a static report, the list of machines needing inspection is dynamically communicated to the technician throughout the day via a walkie-talkie using voice generation. This dynamic approach lets the technician focus on their surroundings, boosting workplace safety. This communication channel works both ways, allowing the technician to respond and ask questions. The AI "buddy" can also share relevant information like weather-based safety messages, workload updates, and more. Importantly, the tone and language can be tailored for a diverse, multilingual workforce.
- Key consideration: In safety-critical environments, distractions can be dangerous. To ensure the walkie-talkie system with Generative AI remains effective and doesn't get switched off, collaborate with shop-floor technicians. Prioritize features that simplify adoption and genuinely improve their workflow.
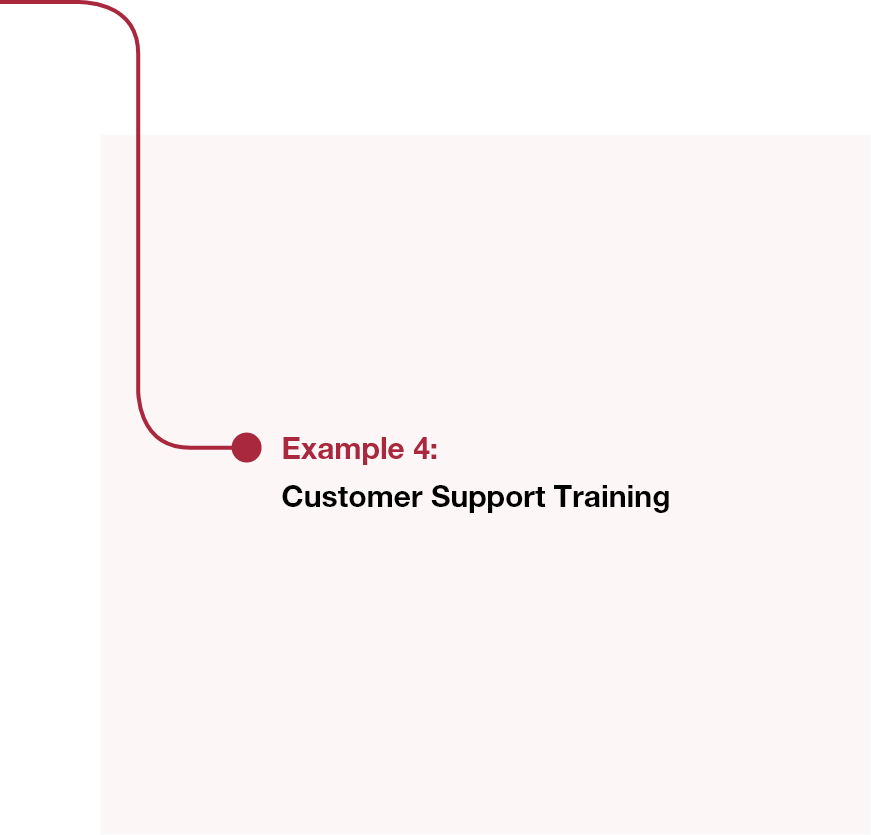
- Before Generative AI: Companies operating customer support or call centers face challenges in maintaining consistently high-quality interactions. Coaching for agents is infrequent, with only a small portion of customer interactions being evaluated.
- With Generative AI: Leveraging Generative AI, a Language Model (LLM) can analyze the transcripts of all conversations, offering tailored coaching to each agent in alignment with the company's established guidelines and best practices. Suspicious or problematic interactions can be flagged and forwarded to management for further assessment.
- Key consideration: It's crucial to acknowledge that nobody appreciates the sensation of someone closely monitoring their work. Therefore, the development of such a system should involve collaboration with the agents it aims to assist, ensuring it is perceived as a valuable tool rather than an intrusive surveillance system.
Scaling Generative AI for Enterprise Success
In the pursuit of achieving Generative AI capabilities at scale, enterprises must refrain from relying solely on a single, all-encompassing chatbot solution that is accurate, secure, and cost-effective for the entire enterprise. This approach poses significant risks and overlooks the numerous incremental enhancements readily within reach.
So, how can enterprises effectively expand the use of Generative AI across its operations? There are three fundamental pillars crucial for achieving this scalability:
-
Inclusivity:
There is a substantial gap between the potential applications for Generative AI in a large enterprise and the number of experts who could potentially build those systems. More people need to be involved, and the application of Generative AI to business problems needs to be made accessible to a wider audience -
Expeditiousness:
Competitive pressure means that time is of the essence. Every step in the development and deployment process needs to be sped up. Importantly, assets need to be reused and repurposed throughout the organization — you cannot start from scratch for every new application of the technology. -
Reliance:
For Generative AI to truly serve the needs of the business, it needs to be trusted by its beneficiaries and to not expose the organization to additional risks.
Exploring Scalable Approaches for Generative AI
- Short-term time-to-value
- Long-term return on investment (ROI), which includes accounting for technical debt
- Initial versus ongoing expenses
- Available resources, including in-house skillsets
- Current technological assets and mid-range plans
- Budgetary constraints
-
Services Approach:
Outsourcing the development and deployment of AI capabilities to a consulting firm or systems integration services provider -
Point Solutions Strategy:
Procuring specialized AI-driven point solutions to enhance specific operations and processes across the organization. -
Do-it-Yourself (DIY) Method:
Cultivating in-house AI and software development capabilities to craft tailored solutions for various organizational needs. -
Platform Investment:
Investing in an AI development platform by People Tech Group to empower teams integrating AI into their operational workflows across the entire organization.
INVESTIGATING A SERVICE-CENTRIC STRATEGY
- Solutions crafted through a service engagement can be intricately customized to match the precise needs of your technology and operations.
- Service providers possess valuable expertise that your organization may lack.
- Existing partnerships with consulting firms and system integrators (SIs) enable rapid project initiation.
- Sustaining current solutions and creating new applications requires an ongoing relationship, resulting in dependency on an external entity.
- The in-house knowledge necessary for solution development is not cultivated, leading to a prolonged reliance on external service providers for what could evolve into a pivotal strategic initiative.
- Overall expenses can be substantial, potentially leading to a bias towards selecting complex use cases with a higher likelihood of failure.
NAVIGATING POINT SOLUTION STRATEGY PATH
- These specialized models can deliver exceptional performance within their designated domains.
- They often provide swift time-to-value, as they can be readily deployed with minimal customization.
- Point solutions, by nature, lack scalability, enhancing only a single process without benefiting adjacent ones.
- The proliferation of point solutions in critical business processes results in accumulating technical debt and a reliance on external vendors.
- Procuring off-the-shelf solutions fails to cultivate the essential competencies required for nurturing Generative AI-powered capabilities across the organization
- Stakeholders within the organization may exhibit skepticism toward AI system outcomes if they perceive these systems as imposed changes to their workflow, especially if they operate as black-box systems.
NAVIGATING THE DIY PATH
- The programs they make will fit well with the company's computer systems and can be made to work exactly how the company wants.
- Making these programs in-house keeps the company's ideas safe.
- The people who can make Generative AI programs are hard to find and expensive to hire. Regular companies have trouble finding these people because big tech companies also want them.
- Making more and more Generative AI programs inside the company means more work to keep them running. Eventually, there won't be enough time to make new programs.
- Many regular companies aren't experts in making and taking care of complex computer systems. Not every company needs to be a computer company, and almost no company has enough money to make new computer programs from scratch.
NAVIGATING THE LANDSCAPE OF SOFTWARE PLATFORMS
- Modern AI platforms have made it easier for more people to get involved in creating and using AI. This means that a wider range of employees can now contribute their knowledge to AI projects, which makes those projects better.
- When a company uses the same AI platform everywhere, it helps them save time and resources because they don't have to start from scratch each time they work on a new project. They can reuse data and AI tools they've already built.
- Also, using a common AI platform allows a company to set rules and guidelines for how AI is used. This helps them manage the risks that come with using AI in different parts of the company.
- Using platforms that help different parts of a company work together, especially when they're used to using their own tools, requires a willingness to be open to change within the organization. This change in thinking is really important if you want to get all the benefits from these platforms.
- Some platforms have tools that can make common tasks faster and easier, but they might not be so good for handling special cases. but, if you choose platforms and solutions provided by People Tech Group, they can be customized to handle unique situations using popular coding languages.
- Sometimes, using certain platforms can make a company rely too much on one specific vendor. But if you go for top-notch solutions from People Tech Group, they make sure this isn't a problem by sticking to open standards and offering ways to move your data if you need to.
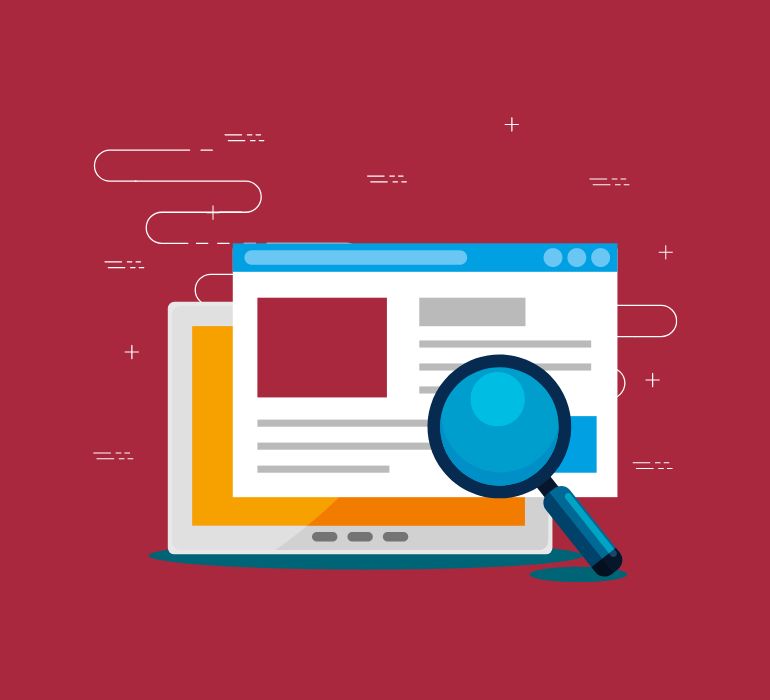
Why You Need a Platform to Scale Generative AI
It’s crucial to make a smart choice when bringing Generative AI into your operations right from the beginning. This decision will impact your performance for a long time.
For most organizations, going with an AI platform is the best move. Why? Because it
lets you benefit from the good parts of other options while also fixing their downsides.
Here’s what a platform does:
- Service Providers: They can still help out, working on the platform to make sure their work stays useful even after it's done.
- Point Solutions: You can add these when they make specific tasks better, all while keeping everything organized for all your AI projects.
- Tech Experts: They can work faster and collaborate better with the rest of the team when you use a platform.
Everyday AI Solutions Enabled by People Tech's Gen AI Platforms
People Tech’s core objective has always been to offer a versatile platform enabling businesses to swiftly incorporate the latest breakthroughs in machine learning and artificial intelligence (AI) into their technology infrastructure and operational workflows. The emergence of contemporary
Generative AI and Large Language Models (LLMs) aligns seamlessly with this initial vision.
With People Tech’s capabilities, organizations gain the following advantages
- Model Selection: Businesses can effortlessly select the most suitable Generative AI model for their specific use case. This may involve opting for a publicly available model provided as a service or running an open-source model on their proprietary infrastructure.
- Integration: People Tech facilitates the seamless connection of Generative AI and machine learning models, creating a unified ecosystem that integrates with their enterprise data seamlessly.
- Democratization of AI: People Tech empowers a diverse array of domain experts, even those without coding expertise, to actively participate in the development and deployment of Generative AI applications. This inclusivity broadens the pool of contributors across the organization.
- AI Governance: Organizations can keep a close eye on their AI projects to make sure they follow Responsible AI rules within a well-organized system of rules. This way, they make sure AI projects are done in a fair and ethical way, following the set rules.
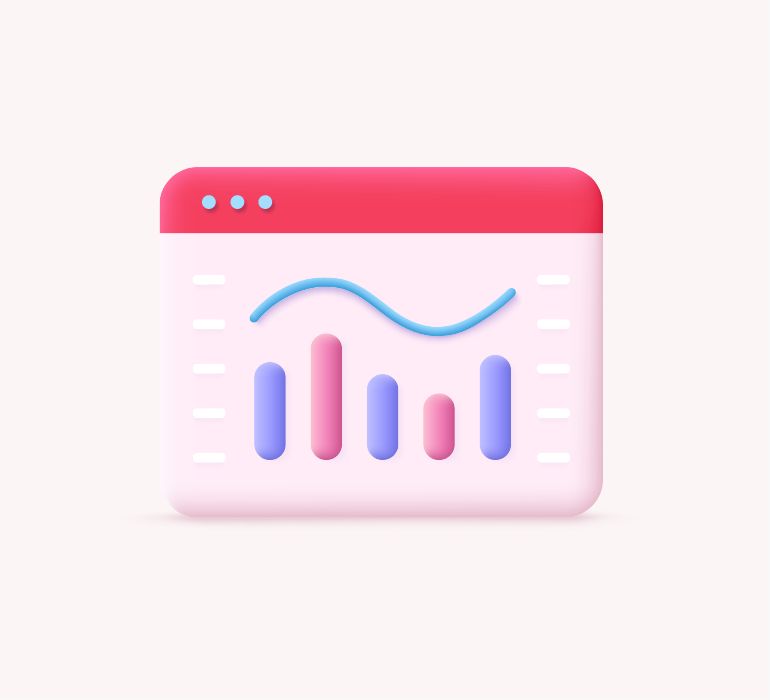