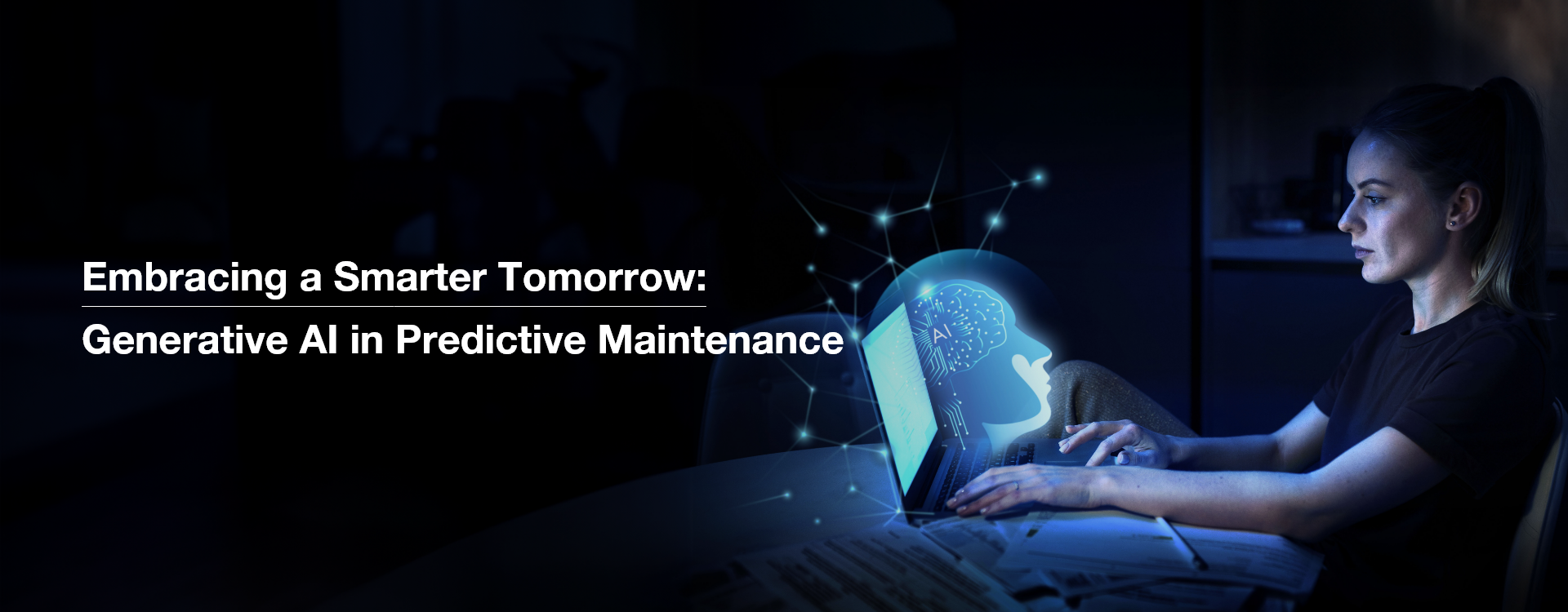
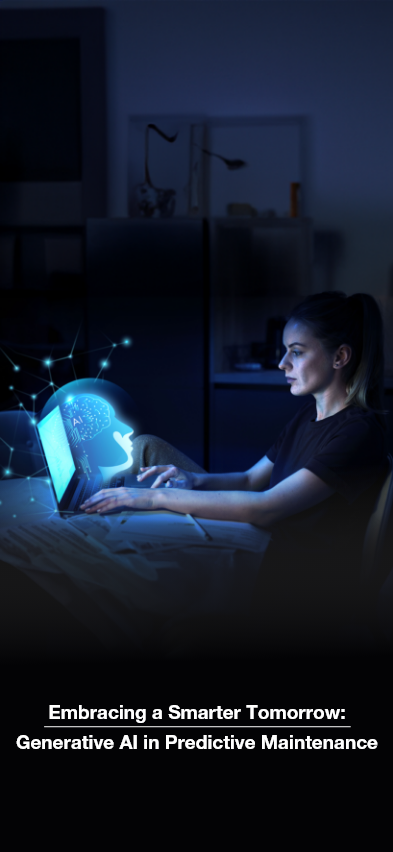
In today’s ever-changing world of industries, the combination of technology and innovation has opened up new possibilities for predictive maintenance. This groundbreaking approach ensures efficiency and reliability, and at the forefront of this transformation is generative AI.
With its ability to analyze a vast amount of data and identify patterns, generative AI is revolutionizing predictive maintenance across various sectors, including IoT, automobiles, and defense.
As we explore the realm of this cutting-edge technology, let’s uncover the challenges, opportunities, and real-world applications that shape the future of maintenance.
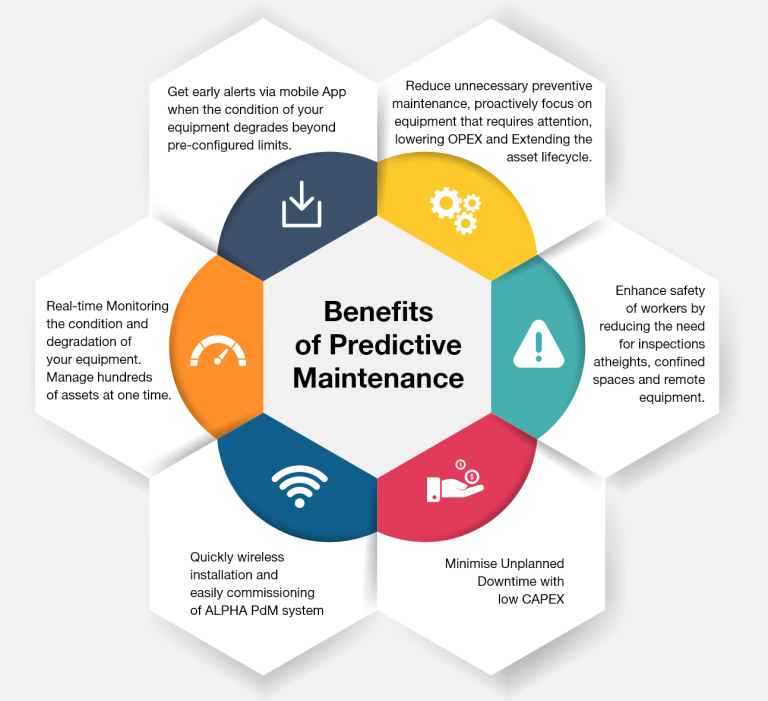
Unveiling the Potential:
Generative AI is reshaping the landscape of predictive maintenance by leveraging its capacity to analyze extensive datasets and recognize patterns. In the realm of IoT, where interconnected devices communicate seamlessly, AI algorithms can access sensor data and decode intricate patterns to predict equipment failures. Just imagine a world where your smart home can anticipate a malfunctioning thermostat or a refrigerator on the brink of breaking down, enabling you to take preemptive measures and avoid inconvenient disruptions.
In the automotive industry, generative models decipher the language of sensors embedded in vehicles. By analyzing the signals emitted by different components, these models anticipate potential failures, allowing for timely intervention. Imagine a situation where your car notifies you of an upcoming issue with the braking system, prompting you to schedule maintenance before a breakdown occurs.
For the defense sector, where reliability is of utmost importance, generative AI ensures that military equipment operates at its highest level of efficiency. By examining sensor data from tanks, aircraft, and communication systems, AI predicts possible malfunctions, offering a strategic advantage by reducing downtime and maximizing operational readiness.
Overcoming Challenges:
However, the implementation of generative AI in predictive maintenance poses certain challenges. In the realm of the Internet of Things (IoT), the vast amount and diversity of data present significant obstacles. Sorting through this flood of data and extracting meaningful insights requires advanced algorithms that push the boundaries of current computational capabilities.
The automotive industry faces a significant challenge due to the intricate nature of modern vehicles. With numerous interconnected systems and sensors, it requires AI models of exceptional accuracy to effectively analyze and identify potential issues.
In addition, there are unique challenges related to stringent security protocols and classified information. The integration process becomes more complex as AI must operate within these restrictions while still providing reliable predictions.
Harnessing Opportunities:
At People Tech Group, we know the opportunities to harness the power of generative models. These models have the potential to improve prediction accuracy by learning from vast datasets.
In the realm of IoT, generative AI can adapt to changing patterns and continuously refine its predictions. In the automotive sector, these models can be trained on diverse datasets that include real-world scenarios, thus enhancing their accuracy. For defense applications, there is an opportunity to create models that can adapt to the ever-evolving dynamics of military operations, serving as a reliable tool for foresight.
By leveraging generative AI models, the automotive industry can address the challenges posed by complex vehicles and improve accuracy in identifying potential issues. Similarly, defense applications can benefit from adaptable models that provide reliable predictions within the confines of stringent security protocols. Overall, incorporating generative AI into these industries offers exciting opportunities for advancement and improved performance.
Furthermore, the iterative nature of generative AI allows for continuous improvement. As more data becomes available and the algorithms evolve, the accuracy of predictions in all three sectors is expected to soar. This iterative learning process is a cornerstone for building resilient and reliable predictive maintenance systems.
Ethical Considerations:
The emergence of generative AI in predictive maintenance raises ethical questions, especially regarding the potential displacement of jobs and concerns about data privacy. While AI streamlines operations and reduces the need for routine maintenance roles, it also creates opportunities for specialized positions, leading to a demand for skilled professionals who can supervise and optimize AI systems.
When it comes to data privacy, the interconnected nature of generative AI necessitates strong safeguards.
Finding a balance between the benefits of predictive maintenance and protecting sensitive information is of utmost importance.
Companies must prioritize transparency and implement strict privacy measures to establish trust with consumers and stakeholders.
Real-World Applications:
Numerous companies are already harnessing the power of generative AI to revolutionize predictive maintenance. In the realm of IoT, smart home devices offered by companies like Nest employ generative models to forecast and prevent malfunctions, guaranteeing uninterrupted service for users.
In the automotive industry, prominent manufacturers such as Tesla utilize AI algorithms to analyze real-time sensor data. By accurately predicting potential issues, Tesla takes proactive measures to address maintenance requirements, thereby enhancing customer satisfaction and prolonging the lifespan of their vehicles.
Within the defense sector, companies like Lockheed Martin rely on generative AI to anticipate equipment failures in military hardware. This ensures that defense systems remain mission-ready, bolstering national security and safeguarding vital operations.
To summarize, the fusion of generative AI and predictive maintenance represents a pivotal moment in the evolution of various industries. Whether it involves predicting equipment failures in IoT devices or optimizing performance in automobiles and defense systems, the applications are truly transformative. Undoubtedly, challenges persist, but the possibilities are abundant, paving the way for a future where efficiency, reliability, and ethical considerations harmoniously coexist.
Talk to our experts to embrace the possibilities, for a smarter tomorrow awaits.